Below is a summary of the abstract you submitted. Presenting author(s) is shown in bold.
If any changes need to be made, you can modify the abstract or change the authors.
You can also download a .docx version of this abstract.
If there are any problems, please email Dan at dar78@pitt.edu and he'll take care of them!
This abstract was last modified on March 4, 2022 at 7:50 p.m..
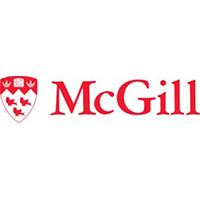
Bacteriophage plaque enumeration is a critical step in a wide array of protocols. The current gold standard for plaque enumeration on Petri dishes is through manual counting. However, this approach is not only time-consuming and prone to human error but also limited to Petri dishes with countable number of plaques resulting in low throughput. We present OnePetri, a collection of trained machine learning models and open-source mobile application for the rapid enumeration of bacteriophage plaques on circular Petri dishes. Our machine learning models were trained using images of plaque assays performed by students in the SEA-PHAGES program. A total of 137 and 4,875 reference objects were used to train the object detection models for Petri dishes and phage plaques, respectively, both having high precision and recall. When compared against the current gold standard of manual counting, OnePetri was ~30x faster. Compared against other similar tools, OnePetri had lower relative error (~13%) than Plaque Size Tool (PST) (~86%) and CFU.AI (~19%), while also having significantly reduced detection times over PST (1.7x faster).